A silent revolution is underway within global financial institutions. AI for financial services has become the linchpin of strategic decision-making, risk management, and customer engagement in the finance sector.
Consider this:
The AI market in fintech is projected to reach $41.16 billion by 2030, growing at 16.5% annually.
60% of financial institutions have already adopted AI technologies, reshaping everything from risk assessment to customer service.
In 2023 alone, the finance sector invested $35 billion in AI, with banking investing the most at $21 billion.
The institutions at the forefront of this AI revolution are rewriting the rules of the game, leaving their less adaptable competitors in a perpetual state of catch-up.
But with great power comes great responsibility—and great challenges.
The ethical implications of AI in finance are profound, touching on privacy, fairness, and transparency issues. Regulatory bodies worldwide are scrambling to keep pace, creating a complex compliance landscape that financial institutions must navigate.
In this article, you’ll learn:
How industry leaders like Capital One and JPMorgan Chase leverage AI
The most impactful AI applications transforming financial services today
Strategies for successful AI implementation
The future of AI in finance
From machine learning algorithms that predict market trends to open banking APIs to AI-powered chatbots revolutionizing customer service, we’ll explore the tools and technologies redefining what’s possible in finance.
Current State of Artificial Intelligence Adoption in Financial Institutions
The current state of AI adoption in the financial industry is characterized by widespread implementation.
According to an EY 2023 Financial Services GenAI Survey, nearly all financial services leaders surveyed reported that their organizations are deploying AI, with 99% of respondents indicating AI adoption.
The long-term perspective is even brighter, as 77% of executives believe that Generative AI (GenAI) will significantly benefit the financial services industry over the next 5 to 10 years.
Leaders particularly see AI’s potential in enhancing customer and client experiences, with 87% predicting improvements in this area.
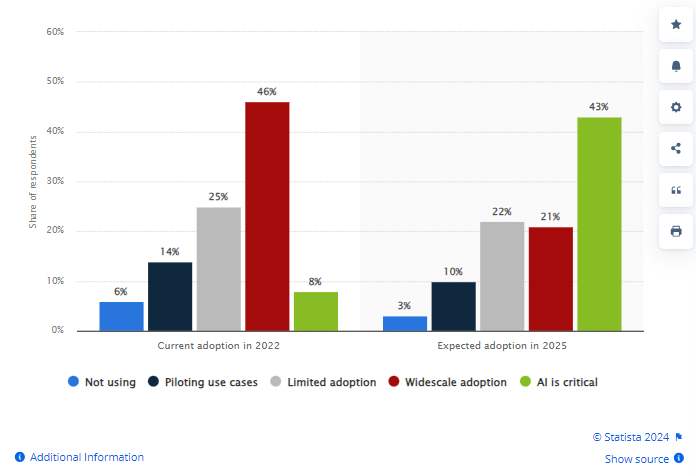
Source: Statista
Industry leaders like JPMorgan Chase and BlackRock have been the pioneers. They have integrated AI deeply into their core operations, leveraging it for everything from risk assessment to investment strategy.
Statistics show that Capital One leads in AI adoption among the largest banks in the Americas and in Europe.
JPMorgan’s Onyx Digital Assets platform, for instance, is part of the broader Onyx ecosystem, which also includes Liink (cross-border payments), JPM Coin (digital currency), and Blockchain Launch (web3 innovations).
Onyx Digital Assets enables financial institutions, asset managers and fintechs to tokenize their financial assets on blockchain, unlocking new utility and mobility for those assets.
The platform allows clients to create tokenized versions of their products, bringing applications of all kinds into the blockchain. It provides infrastructure and resources to support tokenization projects and web3 app development.
Onyx Digital Assets has processed nearly $900 billion in of tokenized U.S. Treasuries as of 2023.
The strategic implications of AI adoption extend far beyond operational efficiencies. AI is reshaping competitive dynamics within the financial services sector, creating new paradigms for customer engagement, risk management, and product innovation.
Institutions that successfully leverage AI are seeing tangible benefits:
Enhanced customer experiences
More accurate risk assessments
The ability to uncover new market opportunities
Looking ahead, the next phase of AI adoption in finance is likely to focus on more sophisticated applications:
Explainable AI for transparent decision-making processes
Federated learning techniques to enhance data privacy and security
Advanced predictive analytics for macroeconomic trend analysis and strategic forecasting
As financial institutions navigate this complex landscape, it’s crucial to recognize that AI adoption isn’t a one-size-fits-all proposition.
The most successful approaches will align AI strategies with the institution’s broader business objectives, organizational culture, and risk appetite.
Leaders must carefully consider how to structure their AI initiatives, balancing centralized oversight with the need for agility and innovation at the business unit level.
Top Applications of AI for Financial Services
1. Machine Learning in Risk Management and Compliance
Machine learning (ML) ability to process vast amounts of data, identify complex patterns, and make real-time predictions has revolutionized how financial institutions approach risk assessment and regulatory compliance.
While traditional risk assessment relied heavily on human intelligence, machine learning algorithms can now analyze many variables simultaneously, far surpassing the capabilities of traditional statistical models.
JPMorgan Chase, for instance, has deployed ML models that can assess credit risk by analyzing financial statements, credit scores, and unstructured data such as news articles, social media sentiment, and macroeconomic indicators.
The impact of ML on compliance processes has been equally profound. Anti-Money Laundering (AML) and Know Your Customer (KYC) procedures, long considered resource-intensive bottlenecks, have been streamlined by applying advanced ML algorithms.
HSBC’s implementation of Google ML-powered AML systems has resulted in a 60% reduction in false positives, significantly reducing the manual workload on compliance teams while improving the accuracy of suspicious activity detection.
This enhances regulatory compliance and contributes to substantial cost savings.
Fraud detection is another area where ML is proving invaluable. Dynamic ML models capable of adapting to new fraud patterns in real time are increasingly supplanting traditional rule-based systems.
AI systems learn customers’ behavior patterns and apply this knowledge during individual transactions, flagging potentially fraudulent activities.
Mastercard’s AI-driven fraud detection system, which analyzes over 75 billion transactions annually, exemplifies this approach. The system has demonstrated remarkable agility in identifying emerging fraud tactics by continuously learning from new data.
Additionally, the application of ML in stress testing and scenario analysis is reshaping how financial institutions prepare for economic uncertainties.
For instance, the Bank of England has three types of stress tests for UK banks and other financial institutions.
Stress testing involves evaluating the resilience of financial institutions under hypothetical adverse economic scenarios, ensuring they have adequate capital to withstand potential financial shocks.
What is Machine Learning in Stress Testing and Scenario Analysis
Enhanced Predictive Capabilities: ML models can analyze vast amounts of historical data and identify complex patterns that traditional models might miss. This enhances the predictive power of stress tests, making them more accurate and reliable.
Scenario Generation: ML can create more sophisticated and realistic economic scenarios by simulating various market conditions and shocks. This allows for a more comprehensive assessment of risks.
Dynamic and Adaptive Models: Unlike static models, ML algorithms can continuously learn and adapt to new data, improving their accuracy over time. This is particularly useful in rapidly changing economic environments.
Efficiency and Speed: ML can automate and accelerate the stress testing process, enabling institutions to conduct tests more frequently and update their risk assessments in near real-time.
This enhanced capability satisfies regulatory requirements and provides strategic insights that inform long-term business planning and risk mitigation strategies.
However, adopting ML in risk management and compliance is not without challenges.
The “black box” nature of some ML algorithms raises concerns about explainability and transparency, particularly in the context of regulatory scrutiny.
Forward-thinking institutions are addressing this by investing in “explainable AI” techniques that provide clear rationales for ML-driven decisions.
Moreover, the potential for bias in ML models, whether due to historical data prejudices or algorithmic design flaws, remains a critical concern that requires ongoing vigilance and mitigation strategies.
2. AI in Trading and Investment
AI trading systems use machine learning algorithms to analyze vast amounts of market data, including historical prices, volumes, and sentiment analysis.
This helps in identifying patterns and trends, which are used to make informed trading decisions.
Quantitative hedge funds and high-frequency trading firms are at the forefront of this revolution.
Renaissance Technologies, long considered the gold standard in quantitative investing, has leveraged AI algorithms to achieve consistent annualized returns of 20% before fees over 30 years.
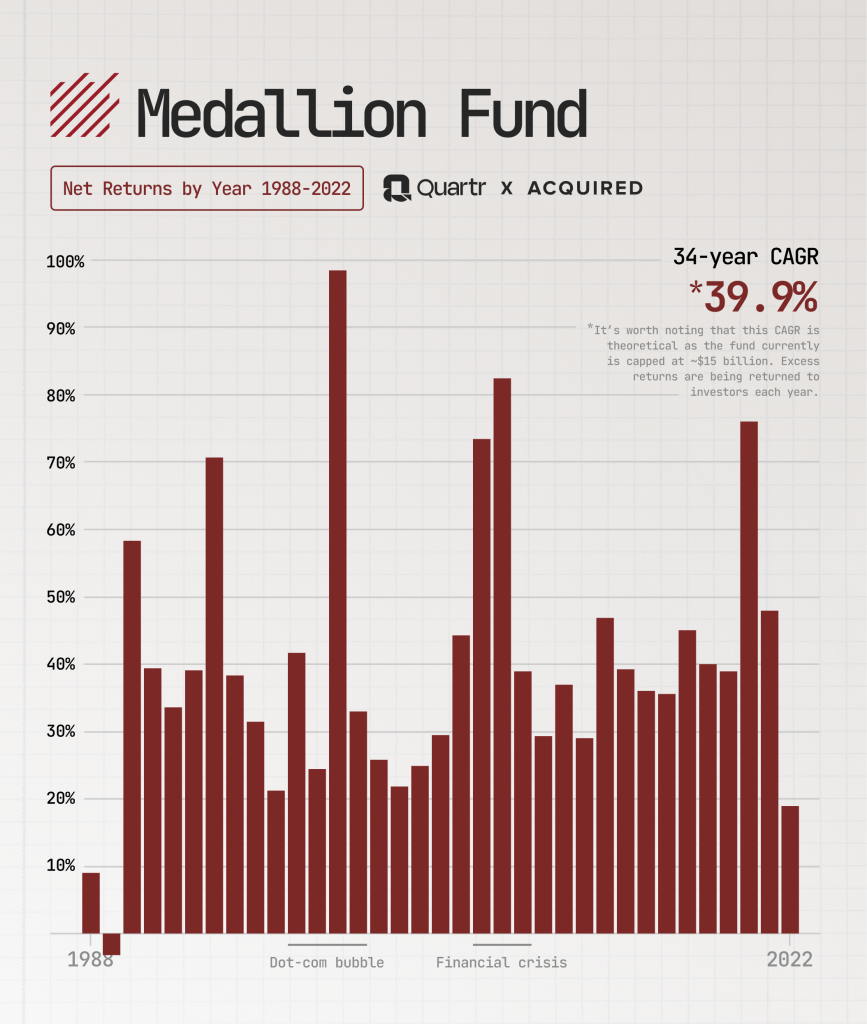
Its quantitative investing approach leverages advanced mathematical models and algorithms like statistical arbitrage, high-frequency trading (HFT), and pattern recognition, including machine learning, to analyze market data and make investment decisions.
This strategy has positioned Renaissance as a leader in the hedge fund industry. The Medallion Fund is considered one of the most successful investment funds in history, achieving remarkable returns far exceeding traditional investment strategies.
Large institutional investors are also increasingly adopting AI to enhance their investment strategies.
BlackRock, the world’s largest asset manager, utilizes its AI engine, Aladdin, to process millions of data points daily, informing investment decisions across $21.6 trillion in assets.
Aladdin uses computers to analyze global economic data, stock market prices, and numerous other economic factors.
It takes into account sudden changes in government, weather conditions, or possible disasters when evaluating portfolios.
This AI-driven approach enables BlackRock to offer sophisticated risk management and portfolio optimization services, solidifying its position as an industry leader.
The impact of AI extends beyond traditional asset classes.
In cryptocurrency markets, where volatility and 24/7 trading present unique challenges, AI-powered trading bots have become ubiquitous.
These algorithms can execute trades based on pre-defined strategies, react to market movements in milliseconds, and even adapt their strategies based on market conditions.
Natural Language Processing (NLP) has emerged as a powerful tool for sentiment analysis and news-based trading strategies.
Firms like Two Sigma and Man Group are utilizing NLP algorithms to analyze vast amounts of unstructured data from news articles, social media, and corporate filings to gain trading advantages.
These AI systems can detect subtle shifts in market sentiment or identify relevant information buried in financial reports, enabling traders to make more informed decisions and react swiftly to market-moving events.
The democratization of AI-powered investment tools is also reshaping retail investing.
Robo-advisors like Betterment, SoFi, and Wealthfront use AI algorithms to offer personalized portfolio management services at a fraction of the cost of traditional financial advisors.
This technology makes sophisticated investment strategies accessible to a broader audience, potentially disrupting the traditional wealth management industry.
Looking ahead, the integration of AI with other emerging technologies promises to revolutionize trading and investment further:
Quantum computing could exponentially increase the processing power of complex financial modeling and risk assessment.
Blockchain technology and AI could enhance transparency and efficiency in trade execution and settlement processes.
Advanced natural language generation (NLG) systems could automate the creation of detailed investment reports and market analyses, freeing human analysts to focus on higher-level strategic decisions.
However, as sophisticated AI tools become more prevalent, there is a risk of creating a two-tiered market where AI-equipped investors have a significant advantage over those without access to such technologies.
This could lead to decreased market efficiency and liquidity in the long run.
3. AI in Personal Finance Industry
Integrating Artificial Intelligence into personal finance fundamentally reshapes relationships between financial institutions and retail customers.
This transformation represents a paradigm shift in how financial services are delivered, consumed, and valued in retail banking.
AI-powered robo-advisors and personal financial management (PFM) tools are at the forefront of this revolution.
Firms like Wealthfront and Betterment have leveraged AI algorithms to democratize access to sophisticated investment strategies traditionally reserved for high-net-worth individuals.
These platforms analyze individual financial situations, risk tolerances, and goals to create and manage personalized investment portfolios.
The impact has been substantial: As of 2023, Wealthfront manages over $55 billion in assets for approximately 735,000 clients, demonstrating the growing consumer appetite for AI-driven financial advice.
Traditional banks are not far behind in this AI-driven transformation.
JPMorgan Chase has implemented AI-driven virtual assistants that utilize NLP to manage a significant volume of customer interactions, enhancing efficiency in customer service operations.
This AI-driven approach has improved operational efficiency and significantly enhanced customer satisfaction scores.
Predictive analytics, a key application of AI in personal finance, enables financial institutions to offer proactive, personalized financial advice at scale. Capital One’s ENO, an AI-powered chatbot, utilizes AI and natural language processing to interpret user inquiries and provide relevant information to customers.
This level of personalization, delivered in real-time, fosters deeper customer relationships and drives customer loyalty in an increasingly competitive banking landscape.
The impact of AI extends to credit decisions, an area traditionally fraught with inefficiencies and potential biases.
Upstart, a lending platform, utilizes machine learning algorithms to approve more borrowers than traditional models.
This demonstrates AI’s potential to expand financial inclusion while maintaining robust risk management practices.
The future of AI in personal finance promises even more transformative applications:
Emotion AI could analyze voice patterns and facial expressions during financial consultations, enabling more empathetic and effective financial advice.
Advanced predictive models could forecast individual financial crises before they occur, allowing for preemptive interventions.
AI-powered financial coaching systems could provide continuous, personalized guidance, adapting to changing life circumstances and financial goals in real time.
4. Advanced Data Analytics and Predictive Modeling
It’s 2025, and you’re the CEO of a major financial institution.
You’re about to decide to expand into a new market. In the past, this would have involved months of research, countless meetings, and a fair amount of gut instinct.
But today, you have a different approach.
You turn to your AI-powered Advanced Analytics Dashboard. Within seconds, it presents a comprehensive analysis:
Market growth projections based on complex economic indicators
Potential risks, including geopolitical factors and climate change impacts
Competitor analysis, including predictive models of their likely responses
Optimal entry strategies tailored to your institution’s strengths
This is the reality that advanced data analytics and predictive modeling are bringing to financial decision-making.
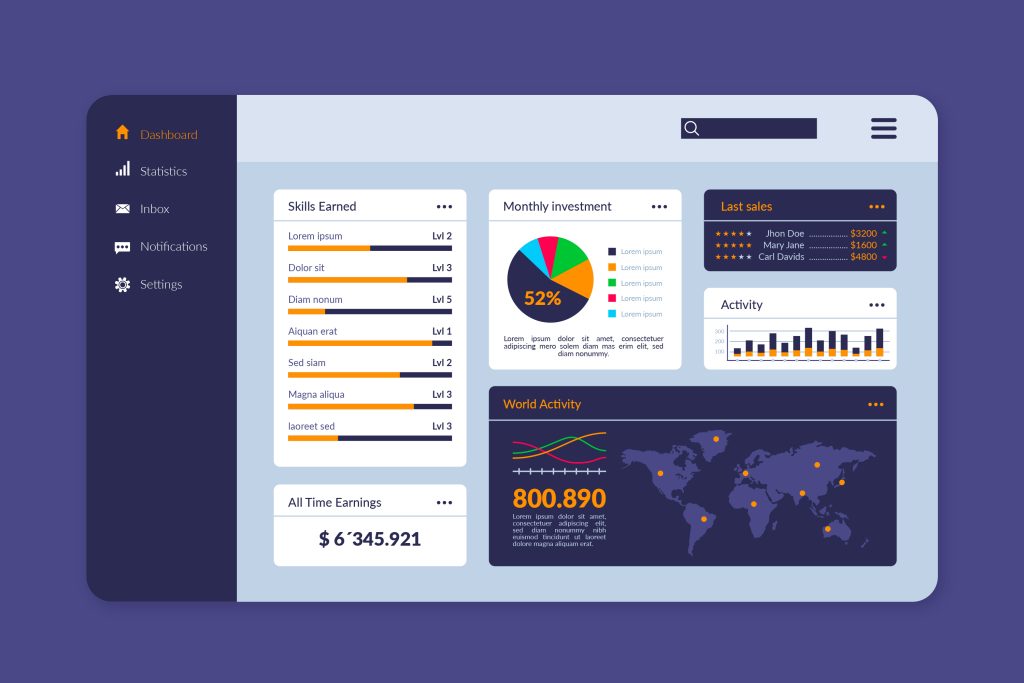
Take JPMorgan Chase’s COiN platform, for instance. It doesn’t just analyze financial data; it combs through millions of documents, extracting key information and identifying patterns that human analysts might miss.
But the real power of these technologies lies in their predictive capabilities.
Goldman Sachs’ Marquee platform is a prime example. It doesn’t just provide data. It offers clients AI-driven insights into market trends, helping them stay ahead of the curve.
Marquee offers a range of services and products, including visual analytics, risk analytics, and trading capabilities.
It provides clients with access to Goldman Sachs’ SecDB pricing and risk system, proprietary research, and execution capabilities.
The impact extends far beyond the boardroom.
In retail banking, predictive models are revolutionizing customer experience. Bank of America’s AI-powered virtual assistant, Erica, doesn’t just respond to customer queries. It anticipates customer needs, offering personalized financial advice based on spending patterns and life events.
This level of personalization, once a pipe dream, is now the industry standard.
Since its launch in 2018, Erica has engaged in over 2 billion client interactions.
However, with great power comes great responsibility. These technologies have profound ethical implications. As models become more complex, the potential for unintended biases increases.
Financial institutions must grapple with fairness, transparency, and accountability questions in AI-driven decisions.
Moreover, the regulatory landscape is struggling to keep pace.
How do you explain a complex AI model’s decision to a regulator?
How do you ensure compliance when your predictive models are constantly evolving?
These are questions that every financial leader must now consider.
Looking ahead, the convergence of advanced analytics with other emerging technologies promises even more transformative possibilities.
Quantum computing could exponentially increase our predictive capabilities, and blockchain could provide unprecedented data integrity and traceability.
The potential is limitless, but so are the challenges.
5. Hyper-personalization of Financial Services
Imagine a bank that knows you’re planning a home renovation before you’ve even contacted a contractor.
It proactively offers you a personalized loan package with terms based on your financial history, current market conditions, and even the projected value increase of your property post-renovation.
Capital One’s use of machine learning algorithms to analyze customer data and predict future needs is a prime example.
By analyzing customer behavior and interactions, Capital One offers products and services that are tailored to individual needs. For example, if a customer has recently experienced a fraud attempt, the company will not offer a credit card at that time.
The virtual assistant Eno uses natural language processing to understand customer queries and provide personalized financial management assistance without requiring customers to learn banking terms.
As of 2021, 14% of customer interactions with Eno were unrelated to banking, demonstrating the assistant’s ability to engage in natural conversations.
But hyper-personalization goes beyond product recommendations.
It’s reshaping the entire customer journey.
BBVA’s AI-driven app gives customers visibility into their deposits and financial data, offering insights that help them manage their finances more effectively.
This includes features like “Upcoming Expenses” and bank account aggregation services, which help customers anticipate and plan for upcoming financial obligations.
The app also features:
An AI-powered voice assistant, UGI (Universal Gateway Interface), which can perform transactions, provide assistance, and even tell jokes.
Functionalities like Valora View that use augmented reality to simplify buying a home. It lets users point their phone’s camera at a home’s doorway and receive pricing information and other details.
The app includes a budget forecasting engine that uses deep learning techniques to forecast expenses and inflows.
Generative AI Integration. BBVA has partnered with OpenAI to deploy ChatGPT Enterprise licenses among its employees.
Looking ahead, the convergence of hyper-personalization with other emerging technologies promises even more transformative possibilities.
Imagine biometric data detecting stress levels during financial discussions, allowing for more empathetic customer service.
Or consider the potential of augmented reality to provide personalized financial advice in real-time as you shop.
6. AI-Powered Fraud Detection and Cybersecurity
Every transaction, every data point, is a potential vulnerability.
Global losses from fraud reached $485.6 billion in 2023, according to Nasdaq’s 2024 financial crime report.
US consumers reportedly lost nearly $8.8 billion to identity theft and fraud scams in 2022, and the number is estimated to reach $23 billion by 2030.
Visa’s Spring 2024 edition of its Biannual Threats Report found pig butchering scams among the top four payment threats against consumers. The FBI saw over $3.5 billion of reported losses related to pig butchering scams in 2023.
But AI is changing the game.
AI can analyze vast amounts of data, including transactional data, social media activity, and other unstructured data, to identify patterns and anomalies that might indicate fraudulent behavior. This helps in detecting potential fraud early on and preventing losses.
AI-powered predictive models can also forecast the likelihood of fraud based on historical data and real-time insights.
This allows financial institutions to take proactive measures to prevent fraud before it occurs.
Mastercard’s AI-driven Decision Intelligence technology exemplifies this proactive approach.
Launched in 2016, DI is Mastercard’s flagship AI-driven fraud detection solution. It analyzes transaction data in real-time to determine the likelihood of fraud.
DI has been implemented across Mastercard’s global network, which processes over 143 billion transactions annually.
The solution has been shown to increase fraud detection rates by 20% on average, with the potential for up to 300% improvement in some cases.
In 2024, Mastercard launched Decision Intelligence Pro (DI Pro), an enhanced version of its AI-powered fraud detection tool.
DI Pro leverages generative AI to analyze one trillion data points in less than 50 milliseconds, providing real-time fraud risk assessment.
Initial modeling indicates that DI Pro can boost fraud detection by 20-300% and reduce false positives by over 85%.
However, as AI systems become more sophisticated, so do the threats they face. We’re entering an era of AI-versus-AI in cybersecurity.
Cybercriminals now deploy machine learning algorithms to:
Probe for weaknesses and orchestrate attacks.
Impersonate legitimate users. For instance, cybercriminals can use AI to mimic human behavior on social media platforms.
Improve algorithms for guessing users’ passwords. This involves analyzing vast password datasets and generating password variations that fit statistical distributions.
Discover vulnerability, particularly through techniques like fuzzing.
Automate repetitive and error-prone security tasks, such as network log analysis, threat analysis, and vulnerability assessment.
This arms race is driving innovation at an unprecedented pace.
The implications extend beyond individual institutions. As financial systems become more interconnected, the security of one becomes the security of all.
Looking ahead, the convergence of AI with other emerging technologies promises even more robust security measures.
When paired with AI, Quantum encryption could create virtually unbreakable security protocols.
Blockchain’s immutable ledger, enhanced by AI-powered smart contracts, could revolutionize transaction verification and fraud prevention.
How Do You Implement AI in Financial Services?
Implementing AI in financial services is a strategic transformation that touches every aspect of your organization.
The journey from concept to execution is complex, but the right approach can be a powerful catalyst for innovation and growth.
1. Start with Strategy, Not Technology
The first step in AI implementation is not selecting a vendor or hiring data scientists. It’s defining your strategic objectives.
What specific business problems are you trying to solve?
Are you looking to enhance customer experience, streamline operations, or improve risk management?
Your AI strategy should align closely with your overall business strategy.
JPMorgan Chase’s approach exemplifies this strategic alignment. The bank’s CFO, Jeremy Barnum, said that the bank is against “chasing shiny objects in AI.”
And to show the preparations and priority the company was placing on AI, in June 2023, the bank hired Teresa Heitsenrether as its chief data and analytics officer to lead AI adoption across the company.
The bank’s AI implementation is focused on enhancing operational efficiency and risk management.
That’s how you should also approach it.
2. Build Your Data Foundation
AI is only as good as the data it’s fed. Many financial institutions find their data siloed, inconsistent, or incomplete.
Before diving into AI implementation, invest in building a robust data infrastructure.
Capital One’s journey is instructive here. They spent years consolidating data across their organization and migrating to a cloud-based infrastructure before launching their AI initiatives.
The company also migrated its operations to the cloud, using Amazon Web Services (AWS) as its primary provider. It has more than 2,000 applications in AWS.
Capital One says that shorter development times on AWS allow them to incorporate customer feedback into new products and features in weeks.
3. Choose the Right Operating Model
There’s no one-size-fits-all approach to AI implementation.
Your operating model should reflect your organization’s culture, size, and specific needs. Consider these models:
Centralized: A single AI team serves the entire organization.
Federated: Central AI team sets standards, while business units have their own AI teams.
Distributed: AI capabilities are embedded within each business unit.
Goldman Sachs opted for a federated model, with a central “AI first” team that collaborates with embedded AI teams in each business unit.
This approach allows standardization and knowledge sharing while maintaining the agility to address unit-specific needs.
It also allows for developing and deploying AI solutions tailored to specific business needs while ensuring centralized oversight and coordination.
4. Invest in Talent and Culture
Implementing AI requires more than just hiring data scientists. You need to build cross-functional teams that blend AI expertise with domain knowledge.
Moreover, fostering an AI-friendly culture across your organization is crucial.
HSBC’s approach to talent development is noteworthy. They’ve implemented an AI training program for all employees, not just technical staff.
The program focuses on upskilling and reskilling employees to ensure they have the right skills to work with AI. This includes training in AI technical skills, such as machine learning and data analysis, as well as soft skills like collaboration and communication.
5. Start Small, Scale Fast
While your AI strategy should be ambitious, your implementation should start with manageable, high-impact projects.
This approach lets you demonstrate value quickly, learn from experience, and build momentum.
Focus on high-impact projects and ensure that the initial AI implementation delivers tangible benefits and demonstrates AI’s value to stakeholders.
This helps secure ongoing support and investment for AI initiatives.
6. Address Ethical and Regulatory Considerations Early
AI implementation in finance comes with unique ethical and regulatory challenges.
Addressing these proactively and establishing clear governance structures and ethical guidelines from the outset is crucial.
Transparency: Be transparent about when customers interact with an AI system instead of a human agent. Avoid deception.
Bias: Ensure AI systems are trained on diverse, representative data to avoid perpetuating human biases.
Privacy: Protect customer data privacy and be clear about how data is collected and used.
Accountability: Establish clear lines of accountability for AI-driven decisions and actions.
There are also regulatory considerations:
Data Privacy Laws: Comply with relevant data privacy regulations, such as GDPR, CCPA, etc., that govern the collection and use of customer data.
AI-Specific Regulations: Stay up-to-date on emerging AI-specific regulations and guidelines in your industry and region.
Explainability: Be prepared to explain how AI systems arrive at decisions, especially for high-stakes use cases.
Human Oversight: Maintain appropriate human oversight of AI systems, especially for sensitive customer interactions.
7. Measure, Learn, and Iterate
AI implementation is not a one-time project but an ongoing process of refinement and expansion.
Establish clear metrics for success and be prepared to iterate based on results.
Integrate AI with CRM systems to comprehensively view customer interactions and preferences.
This helps you understand customer behavior and tailor your AI systems accordingly.
You can also leverage predictive analytics to analyze customer data to predict future behavior and preferences. This helps you proactively address potential issues and improve customer satisfaction.
AI Ethics and Governance in Financial Services
AI ethics and governance in financial services are crucial to ensure the responsible development and deployment of AI technologies, particularly in customer service.
The ethical implications of AI in finance are business-critical considerations that can make or break an institution’s reputation, regulatory compliance, and, ultimately, its bottom line.
The Stakes Are High
Missteps in AI ethics can result in substantial financial penalties, legal challenges, and irreparable damage to an institution’s reputation.
The cost of neglecting ethics in AI development and deployment far outweighs the investment required to implement robust ethical frameworks from the outset.
Fairness and Bias
If not carefully designed and monitored, AI systems can perpetuate or even exacerbate existing biases.
The challenge is twofold: ensuring that AI models don’t discriminate based on protected characteristics and addressing the more subtle issue of proxy discrimination, where seemingly neutral factors can serve as proxies for these protected characteristics.
Financial institutions must implement rigorous testing frameworks to detect and mitigate bias at every AI development and deployment stage.
This includes diverse data sampling, regular equity audits, and the use of explainable AI techniques to understand how decisions are made.
Transparency and Explainability
The “black box” problem becomes increasingly significant as AI systems become more complex.
Financial institutions must be able to explain AI-driven decisions to customers, regulators, and their boards.
Prioritizing transparency and explainability in AI systems is a business necessity that can enhance trust and facilitate regulatory compliance.
Data Privacy and Security
AI systems in finance often require vast amounts of sensitive personal and financial data.
Safeguarding this information is both an ethical imperative and a business necessity.
Institutions must implement comprehensive data governance frameworks that include privacy-preserving AI techniques to balance innovation and privacy protection.
Governance Frameworks
Effective AI governance is about channeling innovation responsibly, not stifling it.
A robust governance framework should encompass everything from data management and model development to deployment and monitoring.
This often requires establishing dedicated AI ethics committees that include technologists, ethicists, legal experts, and business leaders.
Regulatory Compliance
The regulatory landscape for AI in finance is evolving rapidly, with new frameworks and guidelines emerging globally.
Financial institutions must comply with current regulations and anticipate future developments.
This requires a proactive approach to regulatory affairs, working closely with regulators to shape emerging AI policies while ensuring internal practices remain ahead of regulatory curves.
The Path Forward: From Compliance to Competitive Advantage
The true leaders in AI ethics and governance will view these considerations not as constraints but as opportunities for innovation and differentiation.
Financial institutions can unlock new customer loyalty and market leadership levels by building trust through ethical AI practices.
Ethical AI practices can open new markets, drive innovation in financial inclusion and sustainable finance, and create new revenue streams.
Future Outlook for AI in Financial Services
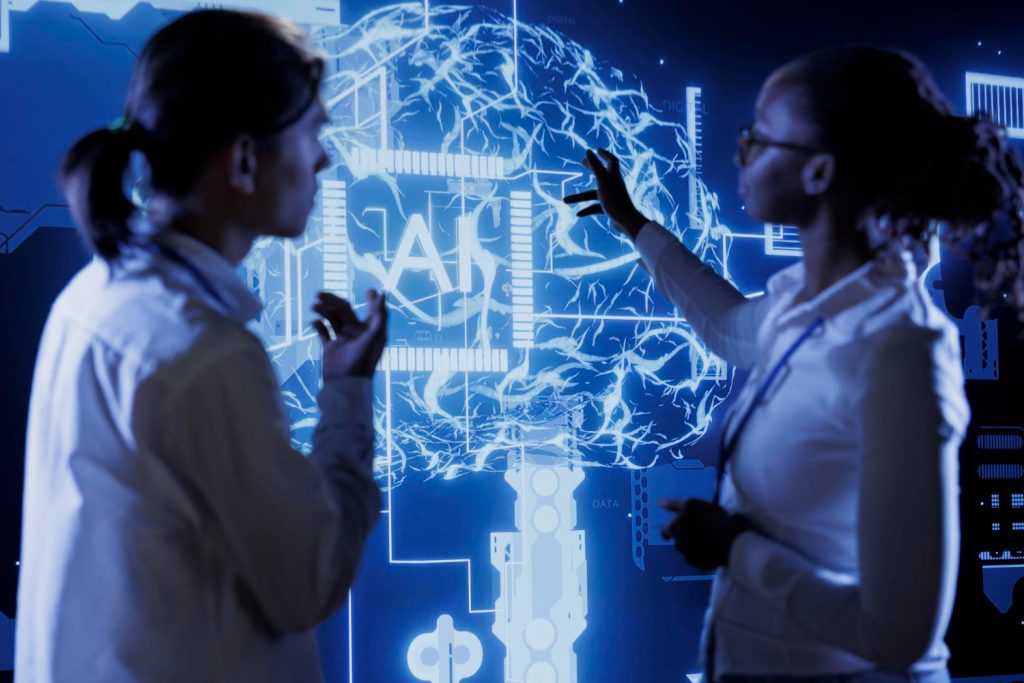
The coming decade will likely witness a paradigm shift in how financial services are conceived, delivered, and consumed. For financial leaders, understanding and anticipating these trends is a strategic imperative.
Here are some of the future technologies to expect in financial services:
1. Generative AI: Beyond Prediction to Creation
Generative AI refers to AI systems that create new, original content based on training data. Unlike traditional AI, which focuses on pattern recognition and prediction, generative AI can produce text, images, code, and even financial models.
According to The McKinsey Global Institute (MGI), it’s estimated that across the global banking sector, gen AI will add between $200 billion and $340 billion in value annually — 2.8 to 4.7% of total industry revenues — through increased productivity.
In financial services, generative AI can analyze vast amounts of financial data, market trends, and economic indicators to generate:
Personalized financial advice and product recommendations
Detailed market analysis reports and forecasts
Synthetic data for model testing and development
Automated regulatory compliance documentation
What to expect: We anticipate generative AI to revolutionize areas like:
Risk assessment: Generating complex risk scenarios for stress testing
Product development: Creating tailored financial products for niche markets
Customer interaction: Powering hyper-personalized chatbots and virtual financial advisors
Generative AI can dramatically enhance productivity, personalization, and innovation in financial services.
It has the potential to reduce costs, improve customer experiences, and uncover new business opportunities that human analysts might overlook.
2. Blockchain Integration
Blockchain is a distributed ledger technology that enables secure, transparent, immutable record-keeping without a central authority.
In finance, the integration of blockchain and AI in finance creates powerful synergies:
AI algorithms can analyze blockchain data to detect patterns, anomalies, and potential fraud
Smart contracts on blockchain can be made more intelligent and adaptive using AI
AI can optimize the consensus mechanisms and improve the efficiency of blockchain networks
What to expect:
More secure and efficient cross-border payments and settlements
Enhanced traceability in supply chain finance
Automated, trustless execution of complex financial agreements
Improved regulatory compliance through immutable audit trails
Blockchain-AI integration can significantly reduce operational costs, enhance security, and enable new financial products and services.
It could rebuild trust in financial systems and open up new markets, particularly in areas with underdeveloped financial infrastructure.
3. Regulatory Technology (RegTech)
RegTech refers to using technology, particularly AI, to enhance regulatory processes in financial services.
AI-powered RegTech can:
Continuously monitor transactions and activities for compliance in real-time
Automate regulatory reporting, reducing manual errors and effort
Predict potential compliance issues before they occur
Adapt to changing regulations by updating compliance models automatically
What to expect:
Real-time, continuous compliance monitoring replacing periodic audits
Predictive compliance, where potential issues are flagged and addressed proactively
Regulatory sandboxes leveraging AI to test new financial products for compliance
RegTech can transform compliance from a cost center to a strategic asset. It can reduce compliance costs, minimize regulatory risks, and enable faster innovation by ensuring new products and services are compliant by design.
4. Decentralized Finance (DeFi)
DeFi refers to financial services built on blockchain networks, operating without traditional intermediaries like banks or brokers.
How AI enhances DeFi:
Risk assessment: AI can evaluate creditworthiness in decentralized lending platforms
Liquidity management: AI algorithms can optimize liquidity across decentralized exchanges
Yield farming: AI can dynamically allocate assets to maximize returns in DeFi protocols
Governance: AI can assist in decision-making for decentralized autonomous organizations (DAOs)
What to expect:
More sophisticated DeFi products leveraging AI for risk management and optimization
Increased accessibility of complex financial services to a global audience
New forms of AI-driven, community-governed financial ecosystems
While still evolving, DeFi represents a potential paradigm shift in financial services.
Engaging with DeFi can position financial institutions at the forefront of this transformation, opening new markets and revenue streams.
5. Quantum Computing
Quantum computing harnesses the principles of quantum mechanics to perform infeasible computations for classical computers.
How does it enhance AI in finance?
Quantum machine learning algorithms can process vast amounts of financial data exponentially faster
Complex financial modeling and simulations that are currently impractical become feasible
Optimization problems in areas like portfolio management can be solved more efficiently
What to expect:
Breakthroughs in cryptography, potentially disrupting current security protocols in finance
Ultra-precise risk modeling and financial forecasting
Real-time, global-scale economic simulations for policy-making and strategy
While large-scale quantum computers are still years away, their potential impact on finance is so significant that early preparation and research are crucial.
Financial institutions that are quantum-ready will have a substantial first-mover advantage.
Strategic Imperatives for Financial Leaders
Develop a comprehensive emerging technology strategy that considers the interplay between these innovations.
Invest in pilot projects and partnerships to build capabilities in these areas.
Foster a culture of continuous learning to keep pace with rapid technological advancements.
Engage proactively with regulators to help shape the governance of these new technologies.
Prioritize ethical considerations and trust-building as you deploy these powerful technologies.
The convergence of these technologies with AI is a chance to reimagine the very foundations of financial services.
The leaders who understand and harness these technologies will be the architects of finance’s future.
Conclusion
From risk management and trading to personal finance and regulatory compliance, AI is driving unprecedented changes in how financial institutions operate, compete, and deliver value to their customers.
The integration of AI with emerging technologies like blockchain, quantum computing, and decentralized finance is opening new frontiers of possibility.
These advancements promise enhanced efficiency, improved risk management, and innovative financial products tailored to the needs of an increasingly digital world.
However, with great power comes great responsibility. AI’s ethical implications and governance challenges in finance cannot be overstated. As financial leaders, you must navigate these waters carefully, balancing innovation with responsibility and agility with security.
The future of finance belongs to those who can harness the power of AI not just as a tool but as a strategic imperative. It requires a holistic approach encompassing technology, talent, ethics, and governance.
The institutions that will thrive in this new era will be those that can adapt quickly, innovate responsibly, and maintain trust in an increasingly complex financial ecosystem.
As you stand at this crossroads of technological revolution and financial innovation, one thing is clear: staying informed is crucial for survival and success in the AI-driven future of finance.
How can you ensure that you’re always one step ahead?
Subscribe to our newsletter and gain a competitive edge:
Get exclusive insights from industry experts and thought leaders
Access case studies and best practices from leading financial institutions
Stay informed about regulatory developments and their implications
Discover opportunities for AI implementation and strategic growth
FAQs: AI in Financial Services
How is AI being used in financial services?
AI in financial services is used for risk assessment, fraud detection, algorithmic trading, personalized banking, credit scoring, and regulatory compliance. It enhances efficiency, accuracy, and customer experience across the industry.
How will AI change banking?
AI will revolutionize banking through hyper-personalized services, automated customer support, advanced fraud detection, more accurate risk assessment, and efficient regulatory compliance. It will enable banks to offer faster, more secure, and tailored services.
How are financial services companies using Generative AI?
Financial services companies use Generative AI to create personalized financial advice, automate report generation, develop tailored financial products, and enhance customer interactions through advanced chatbots and virtual assistants.
What is the future of machine learning in finance?
The future of machine learning in finance includes more accurate predictive models, autonomous trading systems, real-time risk management, personalized financial planning, and enhanced regulatory compliance through continuous monitoring and adaptive algorithms.
How is AI used in investment banking?
In investment banking, AI is used for market analysis, deal valuation, client targeting, risk assessment, and algorithmic trading. It helps in processing vast amounts of data to identify investment opportunities and optimize portfolio management.
What is AI financing?
AI financing refers to the use of artificial intelligence in financial decision-making processes. This includes AI-driven credit scoring, automated lending decisions, personalized financial advice, and risk assessment for various financial products.
How does AI improve fraud detection in finance?
AI improves fraud detection by analyzing patterns across millions of transactions in real-time, identifying anomalies humans might miss. It quickly adapts to new fraud tactics, significantly reducing false positives and catching more fraudulent activities.
What ethical concerns arise from AI use in finance?
Key ethical concerns include potential bias in AI decision-making, privacy issues related to data use, the explainability of AI models in critical financial decisions, and the impact of AI automation on employment in the financial sector.
How is AI transforming personal finance management?
AI transforms personal finance management through robo-advisors, automated budgeting tools, predictive financial planning, and personalized investment strategies. It offers individuals more accessible, data-driven financial guidance.
What challenges do financial institutions face in implementing AI?
Major challenges include data quality and integration issues, regulatory compliance, talent acquisition, ethical considerations, and the need for robust governance frameworks. Institutions also struggle with legacy system integration and ensuring AI transparency.